Accessing how infants represent the visual world
Developmental scientists have sought for more than a century to systematically infer the perceptual and cognitive landscape of preverbal infants’ minds from their behavioral responses— such as grasping, fixating, or visual tracking. Looking times have become a behavioral measure of choice, with now-classic paradigms such as preferential looking, habituation, double psychophysics, and violation-of-expectation. Though not without limitations, looking measures have been deployed to assess visual acuity, the differentiation and categorization of visual objects (e.g., recognizing faces), or the cognitive interpretation of visual scenes in different age groups (e.g., pre-grasping infants) and species. In parallel, the development of infant neuroimaging methods has started to reveal the structural organization, cortical functional specificity, connectivity, and neural activity that support infants’ responses to a variety of visual stimuli—such as faces or objects. Jointly, and despite their limitations, these behavioral and neuroimaging tools have already provided invaluable, and often converging insights into such questions as the development of visual acuity, the domain-specificity of infants’ responses to visual objects, or the impact of early experiences.
Recently developed approaches to data analysis, enabled by development of computational capabilities and machine learning tools, provide new and complementary ways to probe infants’ representations of the visual world. For example, encoding models quantify graded associations between visual information and infants’ neural activity. In turn, decoding approaches test whether visual information is represented within infants’ distributed neural patterns, resolved across channels or processing time. Finally, representational similarity analyses quantify the (dis)similarity of infants’ behavioral or neural responses to multiple stimuli, and compare the implied representational geometries with different groups, measures, or models. These initial inquiries have begun to sketch out the developing landscape of the infant visual brain and the extent to which current artificial neural network models of vision may account for them.
Starting points and mechanisms of change in infants’ high-level vision
Artificial intelligence provides a menu of computational frameworks and models to tease apart how infants represent, learn from, and interpret visual information. These ideas are not new, but current measurement and analysis tools have enhanced the capability of developmental science to test these ideas empirically. Existing data already suggest several ways in which current artificial neural networks fall short of capturing how infants’ brains “solve” visual pattern recognition. For example, head-camera datasets have started to document infants’ visual experiences (see also Lisa Oake’s post in this blog), but these datasets reveal clear disconnects: while artificial neural networks typically learn from a few views of very large numbers of exemplars, infants learn from many, mostly unsupervised views of the same few exemplars (i.e., faces and objects). In addition, infants don’t just passively receive all visual input equally, but shape or prioritize it in ways that change over development. For example, young infants tend to track and be exposed to many close, upright, frontal views of a small number of individual faces. Another notable characteristic of infants’ visual learning is that the immature infant brain develops while visual learning occurs. For example, infant acuity is relatively low at birth then develops over the first few years of life, which may facilitate visual recognition learning by initially biasing the visual system towards low-frequency features. While artificial neural networks tend to be trained to solve a discrete problem, infants’ visual learning unfolds in constant interactions (or cascades) with other domains such as motor (e.g., transition from crawling to walking), language (e.g., word learning), and social (e.g., social referencing) development.
The challenges listed above do not necessarily invalidate artificial neural networks as potential models for human vision: rather, they motivate further research on infants’ high-level vision, and suggest that considering experiential, behavioral, and neural data from human infants is necessary to build computational models that better account for the input statistics, implementational constraints, representational means, and computational goals of human vision during its ontogeny. With collaborators including Richard Aslin, Alexis Black, and Lauren Emberson, and with support from the National Science Foundation, my laboratory looks forward to continuing to contribute to this exciting area of research.
Some lessons learned as an early career researcher
Looking back on the ~10 years that have elapsed since I started researching infant development—as a PhD student with Olivier Pascalis and Edouard Gentaz—two small “lessons” stand out. They are not at all original, but perhaps they bear repeating here.
Our field is constantly evolving, and sooner or later there will come a time when learning new concepts or methods becomes necessary to address a question of interest—perhaps an unfamiliar topic, a new computational or statistical skill, a new neuroimaging tool, a new way to think about sampling and representation, etc. Just a little curiosity can go a long way, and many resources exist to help early career scientists learn (such as the “Tools of the trade” series on this blog). Even so, sometimes we need to learn from a different field, self-teach, or otherwise venture outside of well-marked trails. This is OK—the famous piece from Schwartz (2008), on the need to productively embrace ignorance as a researcher, comes to mind.
Science is a social and collective endeavor, a “team sport”. As such, I am continually grateful for the myriad ways in which being part of the research community is a source of much joy, strength, and meaning—whether through current and former collaborations, mentoring early career scientists, receiving formal and informal mentorship, attending the ICIS conferences, or engaging in scientific outreach beyond academia. Yet, as I look to “pay it forward”, I also acknowledge that research practices can marginalize and fall short of the promise that “everyone has the right freely […] to share in scientific advancement and its benefits” (UNHR, Art. 27). Entrenched inequities in access to funding, citations, collaborations, mentoring, conferences, hiring, published findings, equipment, data, etc., are well documented (see blog posts such as this, this, or this one). As researchers, we can act upon these insights to foster inclusivity through our networks and practices; the ICIS Founding Generation Summer Fellowship is a wonderful initiative in that regard.
Acknowledgements
I am grateful for the current funding support of the National Science Foundation (BCS 2122961) and of the College of Arts & Sciences at American University, as well as for the collaborators, mentors, mentees, colleagues, infants, and families with whom I have been lucky to work.
Suggested Readings
Bayet, L., Zinszer, B. D., Reilly, E., Cataldo, J. K., Pruitt, Z., Cichy, R. M., … Aslin, R. N. (2020). Temporal dynamics of visual representations in the infant brain. Developmental Cognitive Neuroscience, 45, 100860. https://doi.org/10.1016/j.dcn.2020.100860
Emberson, L. L., Zinszer, B. D., Raizada, R. D. S., & Aslin, R. N. (2017). Decoding the infant mind: Multivariate pattern analysis (MVPA) using fNIRS. PLOS ONE, 12(4), e0172500. https://doi.org/10.1371/journal.pone.0172500
Jessen, S., Fiedler, L., Münte, T. F., & Obleser, J. (2019). Quantifying the individual auditory and visual brain response in 7-month-old infants watching a brief cartoon movie. NeuroImage, 202, 116060. https://doi.org/10.1016/j.neuroimage.2019.116060
Smith, L. B., Jayaraman, S., Clerkin, E., & Yu, C. (2018). The Developing Infant Creates a Curriculum for Statistical Learning. Trends in Cognitive Sciences, 22(4), 325–336. https://doi.org/10.1016/j.tics.2018.02.004
Vogelsang, L., Gilad-Gutnick, S., Diamond, S., Yonas, A., & Sinha, P. (2019). Initial visual degradation during development may be adaptive. Proceedings of the National Academy of Sciences of the United States of America, 116(38), 18767–18768. https://doi.org/10.1073/pnas.1910674116
Xie, S., Hoehl, S., Moeskops, M., Kayhan, E., Kliesch, C., Turtleton, B., … & Cichy, R. M. (2022). Visual category representations in the infant brain. Current Biology, 32(24), 5422-5432. https://doi.org/10.1016/j.cub.2022.11.016
About the Author
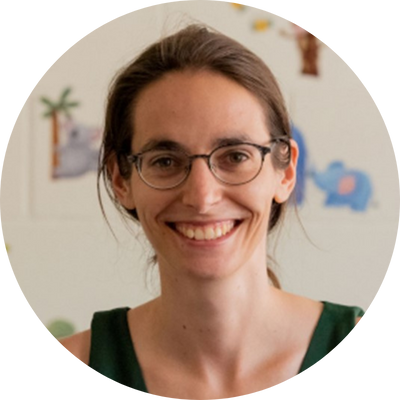
Laurie Bayet
Bayet Lab
Dr. Bayet is a developmental cognitive neuroscientist interested in infant cognitive development and high-level vision. Her lab combines electro-encephalography (EEG), behavioral methods, and computational tools to uncover how infants and young children learn to interpret complex visual objects, including those that are relevant to affective, social communication. Dr. Bayet’s work has been recognized with a Rising Star award from the Association for Psychological Science (APS), and a Distinguished Early Career Contribution Award from the International Congress of Infant Studies. Learn more at: http://www.bayetlab.com/