by Michaela DeBolt and Aaron Beckner
Like many other infant researchers, the pandemic presented us with a dilemma: discontinue data collection or find a w ay to administer studies without increasing exposure risk for families. We decided to conduct studies online so that we could continue our research and as a result, we set up four different studies using Lookit. This allowed us to collect data in a cost- and time-efficient manner. To date, we have collected data from over 500 infants and counting. Currently, we are in the process of analyzing the data from these various studies, but as we look back, there are things that we would have done differently, things that we’re glad we did from the outset, and some general lessons we learned about online testing.
Capturing the in-lab experience
The idea of collecting data completely asynchronously can be anxiety inducing. Many of us wondered whether parents would know how to position their infant or how they would know what to do (or what not to do) if their infant became fussy or disinterested in the screen. These and other concerns led many to pursue synchronous data collection using other platforms such as Zoom. We addressed this problem by creating clear and concise instructional videos for parents. Our goal in making these videos was to capture the “in-person” experience as much as possible, including the rapport building that happens through interactions with research staff as well as the clear instructions that come from those interactions.
The first video parents see in our studies provides a general overview of the study including the purpose and what the parent should expect throughout their “visit”— much like the conversations we would typically have with parents when they arrive in the lab for an in-person visit. The second video parents watch explains the nitty gritty details of what the parent should actually do during the study (e.g., whether and when to close their eyes and a reminder to try not to engage with their infant during the study).
Parents generally reported that participating in our studies was a positive experience so we think that the effort we invested to create these videos was totally worth it.
Coding video data from online studies can be difficult, though not always
The behavioral coding scheme we used for our Lookit data was straightforward. Each infants’ data was coded by two coders — a primary and a secondary coder. The secondary coder only coded a portion of the trials and agreement between the two coders was assessed. Overall, agreement between coders was generally great and when it wasn’t, we included a third coder to resolve any disagreements. A third coder helped resolve disagreements for the vast majority of our data, but periodically all three coders would disagree on a single trial. Surprisingly, these disagreements would occur even for infants whose data was otherwise highly reliable. After ruling out the obvious culprits (i.e., typos), a pattern started to emerge. Despite our best efforts to provide clear instructions, things didn’t always go as planned. Maybe the doorbell rang, a curious sibling pulled at the laptop to see the screen, or a sibling dance party broke out in the background (yes, this actually did happen!). These hiccups are par for the course when collecting data online, but they also made it so that the data from some infants or individual trials were easier to code than others. This was an issue that we expected, but it occurred more frequently than we had hoped. As a result, for some studies we decided to double code all of the data so we could preserve the trials in which agreement was high and exclude single trials in which coders could not reliably agree on the infant’s looking for various reasons (e.g., distractions, momentary loss of interest).
Timing, timing, timing
One issue that several other Lookit researchers discovered, and we helped to confirm, was that there was less precision in the timing parameters than we had initially thought. One advantage of Lookit is that the platform provides extensive information about the timing of specific events, such as when the stimuli become visible on the screen, when the webcam starts recording the infant, or when pauses occur during the experimental sequence. For our studies, we found that
these timestamps often differed from the events observed in the video recording by several hundred milliseconds and that the duration of these timing differences varied across trials and across infants. Lookit has provided a summary of this issue here, but the key takeaway from this discovery is to design studies that will not be adversely affected when timing precision is off by 200 to 500 ms.
Speaking of time, it took a lot of time to train our undergraduate research assistants to code for these projects. Much longer, in fact, than we would typically spend training coders for in-person studies. This was generally due to the fact that we had less control over the quality of video recordings, the distance of the parent from the computer screen, and the potential opportunities for distractions in infants’ environment. This issue was further compounded by the fact that our coders were working remotely and had to use a virtual private network to connect to computers in the laboratory. Despite these limitations, we were able to get a team of coders trained to reliably code for various studies with multiple stimulus sets and study designs. Once we got the ball rolling, things moved relatively quickly and ultimately online testing saved us time in the long-run, as it allowed us to continue testing during the pandemic while requiring minimal effort to administer our studies.
Helping our coders and our babies
Another thing that we generally found helpful was including frequent calibration checks. For all of our studies, we included at least one calibration sequence. This consisted of short, engaging animated video clips that we presented in the locations where our experimental stimuli would subsequently be presented. These calibration animations served two purposes. First, they were included to help disambiguate looking behavior. Our coders reported that calibration checks were incredibly helpful and that they often referred back to them when they were uncertain about the location of a look. Specifically, our coders used the infants’ behavior during calibration to disambiguate (1) looks to the center verses to one side of the screen and (2) looks to one side of the screen from looks away from the screen. Second, these calibration animations were designed to encourage infants to maintain their interest towards the screen. Due to the increased opportunities for distractions, we tried to make our calibration animations as engaging and interesting as possible. Lastly, because babies often shifted positions throughout the study, we recommend including multiple calibration events throughout the study to help capture some of the within-infant looking variability.
Thus, including more calibration animations not only helped our coders, but also helped our babies stay engaged!
Having a plan for data exclusion
As we described earlier, a lot of not-so-surprising distractions occurred in the home while babies participated in our online studies. This meant that we were faced with excluding more individual trials or infants because of unique interference events than we would typically experience in the lab. As one can imagine, the “garden of forking paths” with respect to data exclusion was plentiful. After piloting our online studies, we came up with a list of all the different kinds of things we would consider worthy of data exclusion, and we preregistered these criteria to help streamline the data exclusion decision making process in the future. For example, we reasoned that it was perfectly fine for parents to periodically “peek” at the stimuli during the study, but we would exclude an infant’s data if their parent watched the entire study. We recommend defining exclusion criteria as a lab before analyzing your data because different perspectives, experiences, and ideas can help generate a broader or more practical set of data exclusion criteria to use when it comes time to analyze your data.
A “new frontier” for infant research
In conclusion, we believe that there are many challenges as well as unique advantages to collecting data online. We hope that our reflections are informative, whether you are currently deciding to collect data online, already collecting data online, analyzing results from an online study, or writing up your results for publication. Online testing has provided us with another tool in our methodological “toolbox” and is an exciting new frontier for the field of infant research that will continue to evolve as online testing platforms grow.
About the Authors
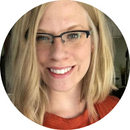
Michaela DeBolt
University of California, Davis
Michaela DeBolt is a Developmental PhD candidate at the University of California, Davis. She studies the development of visual attention in infancy and how individual differences in attention are related to variability in learning.
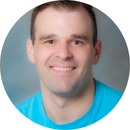
Aaron Beckner
University of California, Davis
Aaron Beckner is a Developmental Psychology PhD candidate working under the supervision of Dr. Lisa Oakes at the University of California, Davis. His research focuses on infant cognition and the development of attention and memory.