Brains are marvels of biological engineering. With 86 billion neurons and trillions of connections, human brains are the most complex structures in the known universe. But where does this structure come from? How does a mass of brain tissue develop into a coordinated, specialized organ capable of perception, cognition, and action?
This question—how the brain acquires its structure—is one of the oldest in science, reflected in the nativist-empiricist debate spanning philosophy, psychology, neuroscience, and biology. Nativists argue that brain structure and core mental skills are innate, encoded in the genome. Empiricists argue that mental skills are products of experience, learned as infants interact with the world. How do we solve this debate and characterize how brains get their structure? Our lab tackles this question by viewing brain development through the lens of protein folding.
The Protein Folding Revolution
Proteins—chains of amino acids that fold into precise shapes—are life’s nano-bricks, assembling and powering almost every structure and process in the body. Like brains, proteins are highly structured, and their structure determines their function. For many years, scientists struggled to understand how proteins acquire their three-dimensional structure from a one-dimensional string of amino acids. This mystery—known as the protein folding problem—persisted for decades.
What finally cracked it? The realization that protein structure is not hardcoded by genes. Instead, proteins fold into shapes that minimize free energy1. The amino acid sequence defines an energy landscape, and the protein finds a stable structure by descending into a local energy minimum. Folding is not deterministic; it’s emergent, shaped by both intrinsic properties (the sequence) and extrinsic conditions (the environment). This was a paradigm shift: the protein folding problem, long thought intractable, became solvable by treating protein folding as an energy-minimization process.
To quantify progress, molecular chemists launched the CASP challenge (Critical Assessment of protein Structure Prediction), a biennial competition to test whether models could predict protein structures based on sequence2. For decades, progress was slow—until 2020, when DeepMind’s AlphaFold2 solved the challenge with breathtaking accuracy3, winning the 2024 Nobel Prize in Chemistry.
Folding Brains, Not Just Proteins
What if brains fold like proteins? We study whether brain development, like protein folding, can be understood as a complex structure emerging through energy minimization (Fig. 1). Brains are not sculpted directly by genes, nor entirely by experience, but by an interaction between the two: brains fold to reduce free energy in structured environments. We propose that brains are space-time fitters that adapt to the spatiotemporal data distributions in prenatal and postnatal environments4. Space-time fitting reduces prediction error, minimizing energy needs of the brain.
To test whether space-time fitters develop core mental skills, our lab created a closed-loop system that combines controlled rearing, artificial intelligence, and virtual reality (Fig. 2):
- Controlled rearing: We raise newborn animals in controlled environments to isolate the specific experiences that shape development.
- Artificial Intelligence: We use generic (blank slate) neural networks as models of brain development. The models have no innate knowledge; they learn (fold) by minimizing prediction error over time, akin to reducing free energy.
- Virtual Reality: We embody the brain models in virtual animals and rear those virtual animals in the same environments as newborn animals. This allows for one-to-one comparisons across developing brains and developing models.
This approach—parallel controlled rearing of animals and models—lets us study brain development as computational folding: a self-organizing process where brains develop structure by minimizing free energy. We test whether complex brain structure and core mental skills emerge from energy minimization, without innate primitives to guide development.
What Have We Found?
Do energy-minimizing models fold into the same structure as newborn brains? Our studies suggest that they do. To study object perception, we reared space-time fitting models in digital twins that mimicked the environments from controlled-rearing studies of newborn chicks5-7. The models developed the same object recognition skills as chicks, simply by minimizing prediction error (free energy) over time. The models learned object constancy—not because they were programmed to do so, but because object perception is a statistical consequence of space-time fitters adapting to a newborn’s visual experiences.
Energy-minimizing models also develop social behavior8,9. We embodied space-time fitters in virtual animals and raised those animals in digital twins of the social environments faced by real animals. Despite lacking innate social knowledge, the models developed rich social behavior, mimicking the collective behavior seen in nature (e.g., shoaling fish, flocking birds, swarming insects). The models also developed social preferences8 (learning to prefer in-group over out-group members) and imprinting behavior9. When energy-minimizing models develop in social environments, they fold into social brains, without an innate social-specific code.
For example, consider a newborn fish raised alongside other fish. Initially, the fish moves aimlessly, bumping into others or drifting away. But over time, the fish learns that predicting the motion of neighbors—by swimming in synchrony or staying close—reduces surprise. Coordinated movement minimizes prediction error and thus free energy, leading the fish to favor shoaling behaviors. These social tendencies are not built in; they emerge as the brain folds to fit the sensorimotor statistics of its social world. In this way, complex social behavior emerges from the same free-energy minimization principle that governs protein folding.
Other core mental skills (e.g., numerical cognition, navigation, motor knowledge, language) also develop in energy-minimizing models10. Brain structure develops when space-time fitters fold to minimize free energy in prenatal and postnatal environments. This finding suggests that a unifying principle—energy minimization—governs biological organization across all scales, from nanometer-sized proteins to meter-sized brains.
From Molecules to Minds
Just as AlphaFold2 cracked protein folding, we believe that developmental science is on the cusp of its own computational revolution. By treating brain development as a problem of folding under constraints—solved by minimizing free energy—we can follow in the footsteps of protein folding and build unified, predictive, and mechanistic models of brain development.
Looking forward, a CASP-like challenge could provide the same catalytic effect for developmental science that CASP did for protein folding. CASP unified the field around shared benchmarks, open data, and a competitive testing framework—dramatically accelerating progress11. A similar brain-folding challenge would allow developmental science to measure whether computational models, raised in the same environments as animals, replicate the developmental outcomes of brains. This challenge would quantify progress and show which models best capture how brains develop over time, driving the field towards a solution for the origins of knowledge.
Figure 1. We propose that proteins and brains develop structure in common ways, by minimizing free energy. Complex brain structure and core mental skills develop spontaneously as brains minimize energy in prenatal and postnatal environments.
Figure 2. Closed-loop system for reverse engineering the origins of knowledge, via controlled rearing studies of animals and models. Modified from ref.12
References:
- Anfinsen, C. B. (1972). The Nobel Prize in Chemistry 1972. The Royal Swedish Academy of Sciences. Nobelprize.org.
- Moult, J., Pedersen, J. T., Judson, R.,. & Fidelis, K. (1995). A large-scale experiment to assess protein structure prediction methods”. Proteins. 23 (3): ii–v. doi:10.1002/prot.340230303
- Jumper, J., Evans, R., Pritzel, A. et al. Highly accurate protein structure prediction with AlphaFold. Nature 596, 583–589 (2021). https://doi.org/10.1038/s41586-021-03819-2
- Wood, J.N., Pandey, L., & Wood, S. M. W. (2024). Digital twin studies for reverse engineering the origins of visual intelligence. Annual Review of Vision Science, 10, 145–70. https://doi.org/10.1146/annurev-vision-101322-103628
- Pandey, L., Wood, S.M.W. & Wood, J.N. (2023). Are vision transformers more data hungry than newborn visual systems? 37th Conference on Neural Information Processing Systems. https://arxiv.org/abs/2312.02843
- Pandey, L., Lee, D., Wood, S.M.W. & Wood, J.N. (2024). Parallel development of object recognition in newborn chicks and deep neural networks. PloS Computational Biology, 20(12): e1012600. https://doi.org/10.1371/journal.pcbi.1012600
- Wood, J.N. (2013). Newborn chickens generate invariant object representations at the onset of visual object experience. Proceedings of the National Academy of Sciences, 110(34), 14000-14005.
- McGraw, J., Lee, D., & Wood, J.N. (2024). Parallel development of social behavior in biological and artificial fish. Nature Communications, 15, 10613. https://doi.org/10.1038/s41467-024-52307-4
- Lee, D., Wood, S.M.W., & Wood, J.N. (2023). Imprinting in autonomous artificial agents using deep reinforcement learning. 37th Conference on Neural Information Processing Systems. Intrinsically Motivated Open-Ended Learning Workshop. https://openreview.net/forum?id=YYndPojV26
- Wood, J. N. (in press). Mini-evolutions as the origins of knowledge. Handbook of Perceptual Development. Oxford University Press.
- Demis Hassabis – Nobel Prize lecture. NobelPrize.org. Nobel Prize Outreach 2025.
- Garimella, M., Pak, D., Wood, J.N., & Wood, S.M.W. (2024). A newborn embodied Turing test for comparing object segmentation across animals and machines. 12th International Conference on Learning Representations.
About the Author
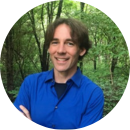
Justin N. Wood
Indiana University Bloomington
Justin Wood is an Associate Professor of Informatics at Indiana University Bloomington. He received his B.A. from University of Virginia and Ph.D. from Harvard University. To characterize the core learning mechanisms in brains, he works at the intersection of developmental psychology, computational neuroscience, virtual reality, and artificial intelligence. Professor Wood has studied the psychological abilities of a range of populations, including human adults, infants, chimpanzees, wild monkeys, and newborn chicks. He has received a NSF CAREER Award, a James McDonnell Foundation Understanding Human Cognition Award, and a Facebook Artificial Intelligence Research Award.